Utilizing Reinforcement Learning for Optimizing Production Sequences in Pharmaceutical Manufacturing
In the high-stakes environment of pharmaceutical manufacturing, efficiency, precision, and compliance are paramount. For Manufacturing IT Managers, leveraging advanced technologies to optimize production sequences can mean the difference between maintaining competitive advantage and falling behind. One such transformative technology is reinforcement learning (RL), a subset of machine learning that uses trial-and-error methods to optimize complex decision-making processes.
By integrating RL capabilities with advanced manufacturing planning systems like PlanetTogether and enterprise solutions such as SAP, Oracle, Microsoft, Kinaxis, or Aveva, pharmaceutical manufacturers can unlock unprecedented levels of operational efficiency.
The Need for Optimization in Pharmaceutical Manufacturing
Pharmaceutical manufacturing is uniquely challenging due to its stringent regulatory requirements, high variability in product demand, and the need to manage complex production lines. Production delays can lead to significant financial losses and, more importantly, impact patient health. Optimization of production sequences ensures that resources are utilized effectively, compliance is maintained, and market demands are met.
Traditionally, production scheduling relies on rule-based systems or linear optimization models. While these methods work well for static environments, they struggle to adapt to the dynamic and uncertain conditions characteristic of pharmaceutical manufacturing. This is where reinforcement learning steps in.
What is Reinforcement Learning?
Reinforcement learning is a type of machine learning where an agent learns to make decisions by interacting with its environment. The agent receives feedback in the form of rewards or penalties based on its actions, which helps it improve its decision-making over time. Unlike traditional optimization techniques, RL excels in environments with high uncertainty and variability, making it ideal for pharmaceutical production scheduling.
Key benefits of RL include:
Adaptability: RL algorithms can adjust to changing conditions in real-time.
Scalability: They can handle complex, multi-variable problems efficiently.
Continuous Improvement: RL systems improve their decision-making with each iteration, enabling long-term gains.
Integrating RL with PlanetTogether and Enterprise Systems
Integrating RL into production planning tools like PlanetTogether and enterprise systems such as SAP, Oracle, Microsoft, Kinaxis, or Aveva provides a powerful framework for optimizing production sequences. Here’s how this integration works and its potential benefits:
Data Ingestion and Preprocessing
PlanetTogether acts as a central hub for manufacturing scheduling and planning data, aggregating inputs such as machine availability, production capacity, and order priorities.
Enterprise systems like SAP or Oracle provide additional layers of data, including inventory levels, supply chain constraints, and financial metrics.
RL algorithms use this data to simulate various production scenarios and evaluate potential outcomes.
Actionable Insights
RL models generate optimized production schedules that align with business goals, such as minimizing downtime, reducing waste, and meeting delivery deadlines.
These insights are fed back into PlanetTogether for execution and into enterprise systems for alignment with broader operational strategies.
Feedback Loops
Real-time feedback from production processes—captured through IoT sensors and manufacturing execution systems (MES)—is fed into the RL model.
This feedback enables continuous learning and adjustment, ensuring that the system remains effective under changing conditions.
Practical Applications of RL in Pharmaceutical Manufacturing
Batch Production Scheduling
RL can optimize batch production schedules to minimize changeover times and ensure compliance with regulatory constraints.
For example, an RL agent might learn to sequence the production of drugs with similar cleaning requirements consecutively, reducing cleaning time and resource usage.
Equipment Utilization
By analyzing real-time data from equipment sensors, RL models can identify the most efficient way to utilize machinery, reducing idle times and increasing throughput.
Supply Chain Coordination
Integrating RL with systems like Kinaxis or SAP allows for synchronized planning across the supply chain, ensuring that raw materials arrive just in time for production while minimizing holding costs.
Risk Management
RL models can incorporate risk factors such as equipment failures or supply chain disruptions into their optimization processes, creating more resilient production schedules.
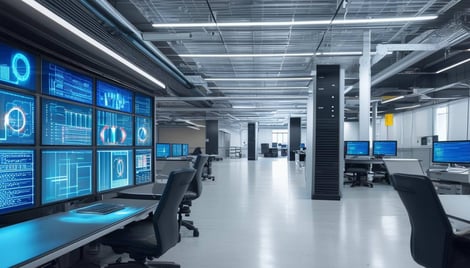
Overcoming Challenges in RL Implementation
While the potential of RL is immense, its implementation is not without challenges:
Data Quality: High-quality, real-time data is critical for RL models to function effectively. Ensuring data accuracy and consistency across systems like PlanetTogether and SAP is a prerequisite.
Computational Complexity: RL models require significant computational resources. Leveraging cloud-based solutions or edge computing can help address this issue.
Change Management: Integrating RL into existing workflows requires buy-in from stakeholders and training for staff. Demonstrating early wins and providing ongoing support can facilitate adoption.
The Role of Manufacturing IT Managers
As a Manufacturing IT Manager, you play a crucial role in driving RL adoption within your organization. Key responsibilities include:
Technology Selection: Evaluating RL tools and ensuring they integrate seamlessly with existing systems like PlanetTogether and SAP.
Data Strategy: Establishing robust data governance practices to support RL implementation.
Stakeholder Engagement: Collaborating with production, quality, and supply chain teams to align RL initiatives with business objectives.
Continuous Monitoring: Ensuring that RL models are regularly updated and fine-tuned to reflect evolving operational conditions.
The integration of reinforcement learning into pharmaceutical manufacturing heralds a new era of efficiency and adaptability. By leveraging the combined capabilities of RL, PlanetTogether, and enterprise systems like SAP or Kinaxis, manufacturers can optimize production sequences, reduce costs, and enhance their ability to respond to market demands.
For Manufacturing IT Managers, the time to act is now. Embracing RL not only positions your organization as a leader in technological innovation but also ensures that you remain resilient in an increasingly competitive and regulated industry.
Are you ready to take your manufacturing operations to the next level? Contact us today to learn more about how PlanetTogether can help you achieve your goals and drive success in your industry.
Topics: PlanetTogether Software, Integrating PlanetTogether, Pharmaceutical Manufacturing, Actionable Insights into Demand Forecasts, Real-Time Feedback Loops, Data Ingestion and Preprocessing
LEAVE A COMMENT