Mastering Inventory Forecasting Models
Production Schedulers play a pivotal role in ensuring that the right products are available at the right time while balancing costs and efficiency. Optimizing inventory management is crucial in pharmaceutical manufacturing.
In this blog, we will look into the intricacies of inventory forecasting models, exploring their importance, implementation, and integration with cutting-edge software solutions like PlanetTogether and leading ERP, SCM, and MES systems such as SAP, Oracle, Microsoft, Kinaxis, and Aveva.
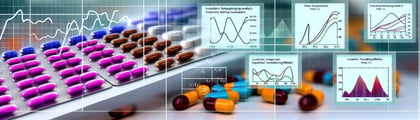
Inventory Forecasting Models
Inventory forecasting models are analytical tools used to predict future demand for products based on historical data, market trends, and other relevant factors. For pharmaceutical manufacturers, accurate forecasting is essential to prevent stockouts, minimize excess inventory, and optimize production schedules.
There are several types of inventory forecasting models, each with its strengths and weaknesses:
Time Series Analysis: This method relies on historical data to identify patterns and trends in demand over time. Techniques such as moving averages, exponential smoothing, and autoregressive integrated moving average (ARIMA) models are commonly used.
Regression Analysis: Regression models establish relationships between demand and other variables such as price, promotions, and seasonality. Multiple regression, linear regression, and logistic regression are examples of techniques used in this approach.
Machine Learning: Advanced machine learning algorithms, including neural networks, random forests, and support vector machines, can analyze vast datasets to uncover complex patterns and make accurate predictions.
Causal Models: Causal forecasting considers external factors that influence demand, such as economic indicators, regulatory changes, and competitor behavior.
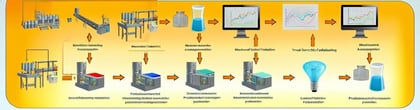
Implementing Inventory Forecasting Models
Implementing inventory forecasting models requires a systematic approach:
Data Collection: Gather relevant historical data on sales, inventory levels, production volumes, and external factors affecting demand.
Data Preprocessing: Cleanse and preprocess the data to remove outliers, handle missing values, and ensure consistency.
Model Selection: Choose the most appropriate forecasting model based on the nature of the data, the forecasting horizon, and the desired level of accuracy.
Model Training: Train the selected model using historical data, adjusting parameters and fine-tuning as necessary to improve performance.
Validation and Testing: Evaluate the model's performance using validation techniques such as cross-validation and out-of-sample testing.
Integration with Production Planning Systems: Integrate the forecasting model with production planning systems like PlanetTogether and ERP, SCM, and MES systems to automate scheduling and inventory replenishment processes.
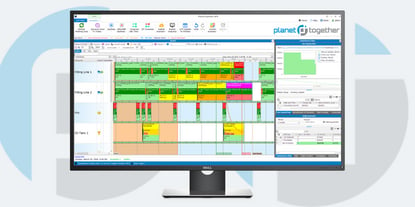
Integration with PlanetTogether and ERP, SCM, and MES Systems
Successful integration between inventory forecasting models and software solutions like PlanetTogether and leading ERP, SCM, and MES systems offers several benefits:
Real-Time Data Exchange: Seamless integration allows for the exchange of real-time data between the forecasting model and production planning systems, enabling timely decision-making and responsive scheduling.
Optimized Production Schedules: By incorporating accurate demand forecasts, production schedulers can create optimized schedules that minimize inventory holding costs while meeting customer demand.
Dynamic Inventory Management: Integration with ERP systems enables automatic inventory replenishment based on forecasted demand, reducing stockouts and excess inventory.
Enhanced Visibility and Control: Production schedulers gain greater visibility into inventory levels, production capacity, and demand fluctuations, empowering them to make informed decisions and adapt quickly to changing conditions.
Improved Collaboration: Integration fosters collaboration between production, planning, and logistics teams, facilitating coordinated efforts to meet customer requirements efficiently.
Best Practices for Effective Integration
To maximize the benefits of integration between inventory forecasting models and software systems, consider the following best practices:
Data Standardization: Ensure that data formats and structures are standardized across systems to facilitate seamless integration and data exchange.
Robust APIs: Choose software solutions that offer robust APIs (Application Programming Interfaces) for easy integration with third-party systems.
Regular Monitoring and Maintenance: Monitor the performance of the forecasting model and software systems regularly, and update them as needed to reflect changes in market conditions and business requirements.
User Training and Support: Provide training and support to production schedulers and other users to ensure they can effectively leverage the integrated systems to improve inventory forecasting and production scheduling.
Inventory forecasting models are indispensable tools for pharmaceutical manufacturers seeking to optimize inventory management and production scheduling. By leveraging advanced forecasting techniques and integrating them with software solutions like PlanetTogether and leading ERP, SCM, and MES systems, production schedulers can achieve greater accuracy, efficiency, and responsiveness in meeting customer demand while minimizing costs and inventory risks.
Embracing these best practices and harnessing the power of integration will position pharmaceutical companies for success in an increasingly competitive market landscape.
Are you ready to take your manufacturing operations to the next level? Contact us today to learn more about how PlanetTogether and integrated scheduling solutions can help you achieve your sustainability goals and drive success in the pharmaceutical industry.
LEAVE A COMMENT