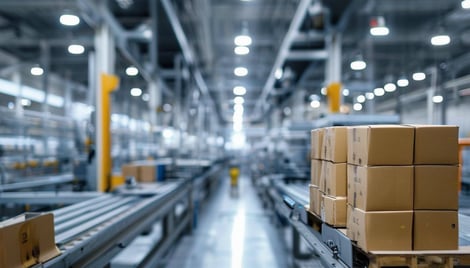
Advanced Workload Balancing via Machine Learning in Packaging Manufacturing
From optimizing production lines to ensuring on-time delivery, Operations Directors must manage a delicate balance of resources, schedules, and priorities. The advent of advanced technologies like machine learning (ML) and their integration into software solutions such as PlanetTogether APS (Advanced Planning and Scheduling) has revolutionized the industry’s approach to workload balancing.
When integrated with enterprise systems like SAP, Oracle, Microsoft, Kinaxis, or Aveva, ML-driven solutions provide transformative benefits that enhance efficiency, reduce costs, and improve overall production outcomes.
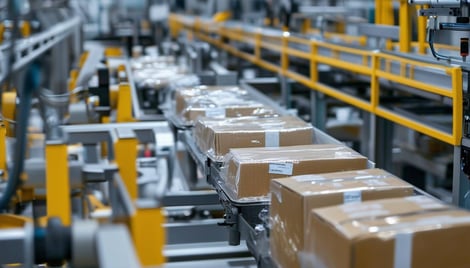
The Complexity of Workload Balancing in Packaging Manufacturing
Packaging manufacturing involves a high degree of complexity, driven by:
Diverse Product Mix: Facilities often handle a wide range of products, each with unique requirements and specifications.
Variable Demand: Fluctuations in customer orders necessitate agile adjustments in production schedules.
Resource Constraints: Limited availability of machinery, labor, and materials can lead to bottlenecks.
Tight Deadlines: Meeting delivery timelines is critical to maintaining customer satisfaction and competitive advantage.
Traditional workload balancing methods often rely on static schedules and manual adjustments, which can lead to inefficiencies, underutilized resources, and missed deadlines. This is where machine learning comes into play.
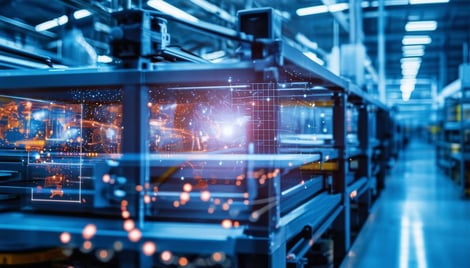
How Machine Learning Transforms Workload Balancing
Machine learning leverages historical and real-time data to predict, analyze, and optimize workload distribution across production resources. Key capabilities include:
Predictive Analytics: ML algorithms analyze past production trends to forecast demand and resource needs.
Dynamic Scheduling: By continuously adapting schedules based on real-time data, ML ensures optimal resource utilization.
Anomaly Detection: ML identifies potential issues—such as equipment failures or unexpected delays—before they escalate.
Scenario Planning: ML models simulate various production scenarios to identify the best course of action under changing conditions.

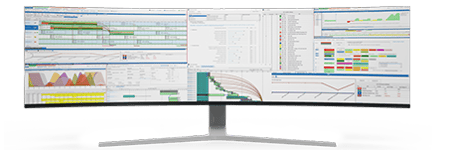
Integrating PlanetTogether with ERP Systems: The Game-Changer
PlanetTogether APS is a powerful tool that enhances production planning and scheduling. When integrated with robust ERP systems like SAP, Oracle, Microsoft Dynamics, Kinaxis, or Aveva, the synergy creates a unified platform for end-to-end operational excellence. Here’s how this integration elevates workload balancing:
Centralized Data Management
Integration enables seamless data flow between PlanetTogether and ERP systems, creating a single source of truth for all production-related information. For example, order details from SAP can be automatically imported into PlanetTogether, eliminating manual data entry and reducing errors.
Enhanced Decision-Making
With real-time data synchronization, Operations Directors gain actionable insights into production performance, inventory levels, and supply chain dynamics. This allows for more informed decision-making and proactive issue resolution.
Improved Collaboration
Integrated systems facilitate better communication between departments, such as procurement, production, and logistics. For instance, a Kinaxis-driven supply chain model can provide PlanetTogether with upstream and downstream visibility, enabling synchronized operations.
Scalable Machine Learning Models
When integrated with advanced ERP systems, PlanetTogether’s ML algorithms can leverage larger datasets for more accurate predictions and optimizations. For example, Aveva’s industrial analytics capabilities can complement PlanetTogether’s ML models by providing insights from IoT-enabled machinery.
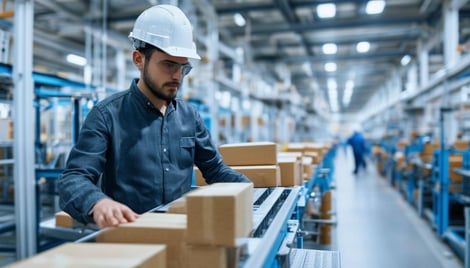
Real-World Benefits of ML-Driven Workload Balancing
The integration of ML-driven tools like PlanetTogether with ERP systems offers tangible benefits for packaging manufacturing operations:
Reduced Downtime
By predicting equipment maintenance needs and dynamically rescheduling tasks, ML minimizes downtime and ensures smooth production flows.
Optimized Resource Utilization
ML algorithms distribute workloads evenly across machines and labor, avoiding overburdening specific resources while ensuring others aren’t idle.
Improved On-Time Delivery Rates
Dynamic adjustments to production schedules based on real-time demand and capacity ensure that orders are completed and delivered on time.
Enhanced Flexibility
With the ability to adapt quickly to changing conditions, ML-driven systems support agile manufacturing, enabling facilities to respond effectively to market fluctuations.
Lower Operating Costs
By optimizing resource usage, reducing waste, and minimizing errors, ML-driven workload balancing significantly cuts operating costs.
Advanced workload balancing powered by machine learning represents the future of packaging manufacturing. By integrating PlanetTogether with ERP systems like SAP, Oracle, Microsoft, Kinaxis, or Aveva, Operations Directors can unlock unprecedented levels of efficiency, flexibility, and resilience. The result is a more agile and competitive manufacturing operation, capable of thriving in an increasingly dynamic market.
Investing in ML-driven solutions is not just about staying ahead of the curve; it’s about redefining what’s possible in operational excellence. For packaging manufacturers, the question is no longer whether to adopt these technologies, but how quickly they can be implemented to deliver maximum value.
Are you ready to take your manufacturing operations to the next level? Contact us today to learn more about how PlanetTogether can help you achieve your goals and drive success in your industry.